0. Referencehttps://arxiv.org/abs/1611.05431 Aggregated Residual Transformations for Deep Neural NetworksWe present a simple, highly modularized network architecture for image classification. Our network is constructed by repeating a building block that aggregates a set of transformations with the same topology. Our simple design results in a homogeneous, mularxiv.org1. WRN- 해당 논문은, Wild Residua..
분류 전체보기
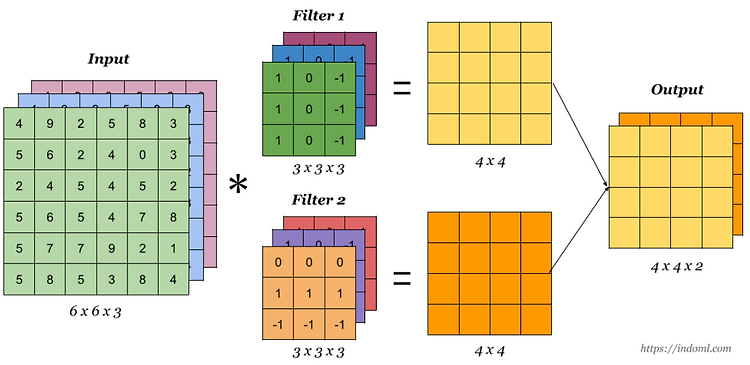
0. Referencehttps://arxiv.org/abs/1610.02357 Xception: Deep Learning with Depthwise Separable ConvolutionsWe present an interpretation of Inception modules in convolutional neural networks as being an intermediate step in-between regular convolution and the depthwise separable convolution operation (a depthwise convolution followed by a pointwise convolution).arxiv.org1. Inception Hypothesis- 우선..
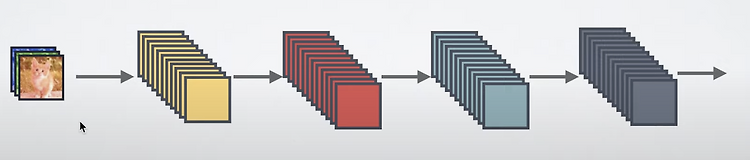
0. Referencehttps://arxiv.org/abs/1608.06993https://www.youtube.com/watch?v=fe2Vn0mwALI&list=PLlMkM4tgfjnJhhd4wn5aj8fVTYJwIpWkS&index=29 Densely Connected Convolutional NetworksRecent work has shown that convolutional networks can be substantially deeper, more accurate, and efficient to train if they contain shorter connections between layers close to the input and those close to the output. In ..
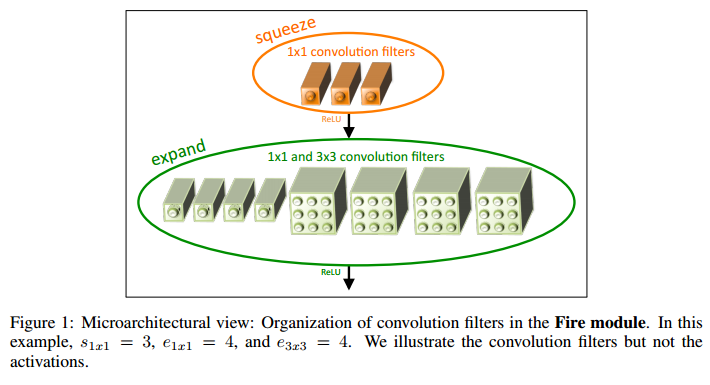
0. Referencehttps://arxiv.org/abs/1602.07360 SqueezeNet: AlexNet-level accuracy with 50x fewer parameters and Recent research on deep neural networks has focused primarily on improving accuracy. For a given accuracy level, it is typically possible to identify multiple DNN architectures that achieve that accuracy level. With equivalent accuracy, smaller DNN architearxiv.org1. Introduction- Squeez..